At Cornell University’s Center for Hospitality Research, one of the main aims is to make research available in a digestible format for those in the hospitality and service industries. A large part of that work involves helping the industry not only collect significant data but to make sense of it in order to make better business decisions.
As part of eCornell’s webcast series, the center’s director, Professor Chris Anderson, joined eCornell’s Chris Wofford for a discussion on data analytics and why industry professionals should adopt a bottom-up approach to data. What follows is an abridged transcript of their conversation.
Wofford: Welcome. Let’s talk about data-driven analytics and what the bottom-up approach means.
Anderson: The first thing to note is that good analytics is not necessarily new. I’ve been in this space for a little more than 25 years now. What’s really happened in the last five to ten years is that analytics have become much more accessible — and with that new accessibility comes lower costs. As a result, it’s become much more widely adopted.
But I think we’ve kind of lost a bit of what I refer to as the bottom-up approach, which is involving those who are critically close to the business itself in the data analytics. You need to have an understanding of where that data came from, what potential variables you’re missing, and how it was sampled. In order to get the most out of data analytics, you need a firm understanding of the business itself and how things should be working towards some sort of outcome. In the opposite scenario, the top-down approach, we let technology tell us what’s going on and we sort of let the data drive the solution.
Wofford: Can you give us a real-world example of what you mean?
Anderson: I come at this historically from the hospitality space, from the demand and pricing side of things. That space to me has always been fascinating because, in order to price and control a hotel or an airline, you really have to have a fundamental understanding of where demand comes from, how the business manages that demand, and what kind of decisions they can make. You really get this deep insight into how you make money.
So for a lot of data analytics, that becomes this core set of skills and once we’re good at it, then we really understand our business well and it brings a lot of opportunities for us.
Wofford: What kinds of data analytics are relevant to the hospitality and service industries?
Anderson: There are three basic forms of data analytics. The first is what we refer to as descriptive, where we’re just describing what has happened or just reporting. It’s kind of a backward view of the world.
Our second is the predictive world, it’s the forward-looking part of analytics where we’re trying to use our insight from reporting to help us look at relationships and make predictions about the future. And then predictive analytics goes one step further and tries to see what factors resulted in us achieving previous metrics, what we might do to impact those and what the future outcome might be.
The third part is prescriptive analytics. Once you understand where you’ve been and have a good sense of how to go forward, then you want to use some tools and techniques to make sure you’re going forward in the profit-maximizing or cost-minimizing sort of way.
It’s about using a set of tools to help us do the best going forward, given the insight that we’ve been able to extract from this both descriptive and predictive framework.
Wofford: What are those tools? What are you looking for within the data?
Anderson: We use things like optimization, where we are looking at making multiple decisions at a time. We use things like decision analysis and programming.
We work on incorporating uncertainty into our decisions. No decision is made out of certainty, so we don’t want to just ignore that. We want to make decisions knowing that there is some uncertainty and once I make one decision I can adjust to those uncertainties and make subsequent decisions.
We use different tools if there’s a lot of uncertainty that’s evolving over time and we might use another set of tools if there’s so much complexity that it’s hard for us to map out how things are all working together.
We think about the starting block as being reporting. Your goal is really to understand how well you’ve been doing, so you’re focused on key performance indicators. How was I pricing? How was my competitor pricing? We are just looking at some of these things together in concert with our backward-looking metrics.
This really lays the groundwork of the predictive part, in which we are trying to understand that these things may be impacting some of our key performance indicators, and we may look at those in different ways.
Even before we can start to do this we’ve got to collect the data, put it in a data warehouse, and have it organized in some sort of centralized way. One of the trickiest parts about this is we have to make sure that we have a lot of integrity around that data. We want to have a secure process from which we can extract, pull and analyze, but we don’t want to necessarily change that underlying structure.
There are a lot of pieces we have to make sure are lined up so that if we have lots of users, they are not going to distract from the quality of that data.
Wofford: In your experience, do you find that most companies have their data in order or when you go to work with them, or do you find you have a lot of work to do right out of the gate?
Anderson: For most organizations, it’s about getting their data house together. It’s often not well organized.
Wofford: So getting that data organized is almost always the biggest challenge?
Anderson: That’s right.
Wofford: Once things are put in order, are we then looking at the predictive component? You mentioned using this to reduce uncertainty – how do we do that?
Anderson: Well, let’s say you are looking at what your sales were last year. That would provide a naive estimate for the next year, right? But while you might be able to take last year’s average, there is a lot of variance around that average. So our goal is to generate a better estimate for the future that has less variance around it, so it’s a more refined guess. We try to make less naive guesses by using information from other attributes that may be impacting sales. If we know those factors going forward, that will help us refine the estimate for whatever that metric is, whether it’s sales or some other key performance indicator. The predictive part is all about reducing uncertainty and we do that through different kinds of relationships.
Wofford: Like competitive analysis, for instance?
Anderson: Right. How my competitor is pricing relative to how I’m pricing. But we have to be cautious because there’s no point in looking at the impacts of relationships unless you know those factors in the future. My sales are a function of how I price and how my competitors price but I don’t necessarily know how my competitors are going to price tomorrow or next week or next year.
Once we’ve got those two parts under our belts – the reporting and the predictive – then we can start to make better decisions going forward instead of just shooting from the hip. And that entails using a lot of these mathematical tools, along with our knowledge, intuition and expertise, to look at some of this complexity.
The prescriptive part is getting us beyond just making obvious logical decisions and trying to look at how things are interconnected. We don’t necessarily jump into this part unless we have our foundations in the information because the prescriptive modeling component is going to need inputs from reporting or inputs from our predictive components. They’re the critical first two steps before we get into part three.
Wofford: And the prescriptive element involves running a simulation in some way?
Anderson: Yes, you could think of it like that. You can think of a hotel trying to set optimal prices to maximize revenue. To do that, the hotel owners have to have some estimate of future demands and ideally some estimate of future price-dependent demand. That estimate of future price-dependent demand from our predictive analysis will then be input into our optimization models to help us formulate those decisions going forward.
Wofford: We hear a lot about things like “text analysis” and other new techniques that help us look beyond simple numeric data. Can you tell me about that?
Anderson: Think of Amazon reviews. We’re selling products on Amazon and we’re looking at what consumers are saying. We have to be cognizant that other consumers are reviewing that content. They’re paying attention to that average review score on Amazon but they’re also actually looking at what people said about the product. So we need to look for keywords and repetition of those keywords.
Yes, I could read all that information manually, but we can now use tools to help us pull up keywords and their frequencies to help us get a sense of what’s going on.
Wofford: I’m guessing this is probably common across all industries at this point.
Anderson: Yes, because now you can review anything. And there’s hardly any business that doesn’t have some sort of online chat service where consumers are typing information. So it’s about trying to look at what questions they’re asking, what problems they’re having with your product and then asking yourself how you can use that data to improve the product.
There’s just so much unstructured text today so we’re trying to look for ways to streamline how we extract insight because we don’t have infinite time to read it. Most of the tools for analyzing text are pretty standardized and most of the algorithms that we can use have been well developed. We’re ten-plus years into things like sentiment analysis so it’s not like we have to reinvent the wheel. There are a lot of off-the-shelf approaches.
Wofford: I’d like to turn to a question from the audience. Peter, who identifies himself as a “non-analytics person” posed this question: “In terms of decisions, I sometimes hear, ‘The numbers don’t support that.’ But it’s often on content that I know has not been marketed. So it seems the decision may be made on numbers that are correct, but that the decision comes from a faulty premise. Is this something you see often?”
Anderson: One of the classic things that I see is that organizations think price is going to impact demand, and they think they are changing prices but what they’re really doing is moving prices seasonally. And when things move together, you can’t really tell the impact of the season versus the price, because those are both adjusting together.
So one of the things we see in that data is that we may not have created the right kind of variance in order to see the outcomes.
Most of us don’t experiment with our business on a regular basis but in order to get insight from data, we have perturb those inputs. It’s just like the science experiments with two petri dishes, where you pour bleach on one and not on the other one to see what kind of bugs grow.
We have to have that experimental mindset when generating this data, because if we’re not making those little perturbations to our business practices, then it’s very hard for us to see how A leads to B because we’ve never manipulated A. Or we’ve only manipulated A at the same time we’ve manipulated B, C and D. If I always drop prices and spend more on marketing together, it’s hard for me to unravel which of those was the driving factor. Our data will not tell us that unless we’re cognizant from the business standpoint of having manipulated those things in such a fashion to generate that variance.
Wofford: So to glean real insight, you’ve got to be willing to take risks?
Anderson: Right. Be like a scientist and do some experimenting. You know, the online world has dramatically changed because of what we call A/B testing. Now it’s so easy to tweak something, so we can do all of these little A/B experiments. It’s very easy to create variances and see the outcome.
Wofford: So in some ways, you describe this as a linear process, but at the same time, it’s not. It’s iterative.
Anderson: It is. One minute to the next. The goal of predictive analysis is to look for robust insight into the future. And that is where, for me, the bottom up approach is critical. Yes, we’re trying to understand your business model but nothing is constant. There could be a new competitor, underlying changes in dynamics or some sort of disruption happening. In order to be robust to those changes, the models that we build from the predictive framework have to be grounded in our business practices.
And that comes from this bottom-up approach, versus just letting the data tell us what’s going on. For me, as a data analyst, it’s always about thinking about my two minute elevator pitch. How do I justify my models and can I clearly explain those models in layman’s terms? If I need to use statistical terminology to explain my insight and my models, that is going to tell me that I’m not necessarily grounded, that I’m relying on the data versus relying on my intuition.
It’s some give and take. You have to go back and forth, but the more bottom-up you are, the easier it is for you to justify models and to communicate those models to other people.
Wofford: I want to thank Chris Anderson for joining us today.
Anderson: Thank you, Chris, this was great.
Want to hear more? This interview is based on Chris Anderson’s live eCornell WebSeries event, A Bottom Up Approach to Data-Driven Analytics and Why We All Need to Be Involved. Subscribe now to gain access to a recording of this event and other Hospitality topics.
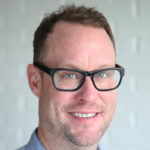
Latest posts by Chris Wofford (see all)
- How Entrepreneurs Think and Behave - November 14, 2018
- Empower Your Team Through Servant Leadership - September 9, 2018
- Cornell’s New Certificate Program Equips Learners with Essential Critical Thinking and Problem-Solving Skills - July 23, 2018